August 5, 2024
This spring, Professor Jonathan Liu received an R01 grant from the National Institute of Diabetes and Digestive and Kidney Diseases of the National Institutes of Health (NIH) to develop computational 3D pathology methods for Barrett’s esophagus risk stratification.
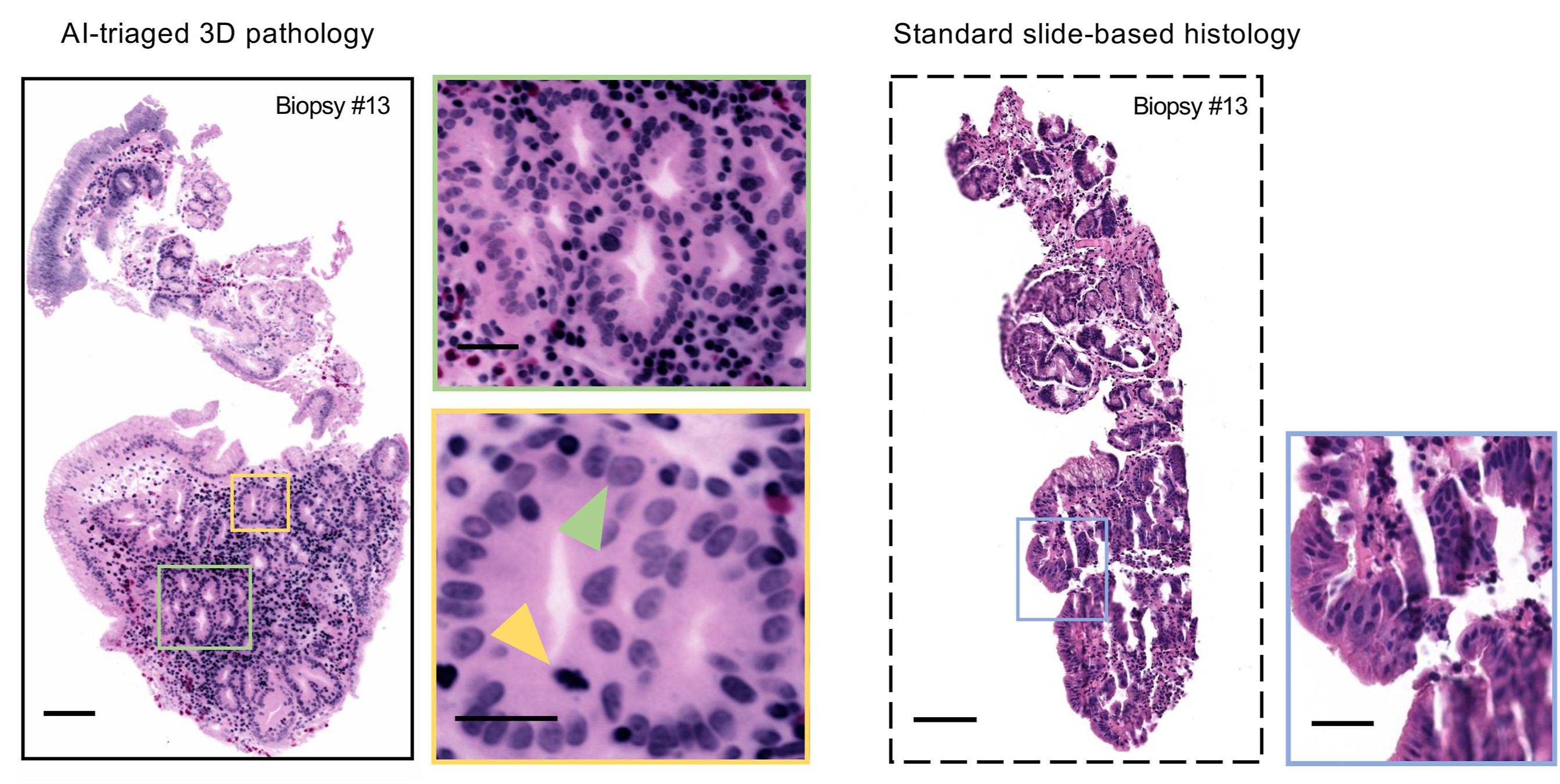
Examples of AI-triaged 3D image sections of a biopsy show how 3D pathology (left) upgraded the diagnosis compared with conventional 2-dimensional methods (right).
The award is $3.65 million over five years. The grant will fund clinical studies using archived tissues from patients with the condition.
After learning how Liu’s team had previously invented 3D pathology methods to assess prostate cancer risk, Deepti Reddi, assistant professor in laboratory medicine and pathology at UW Medicine, contacted him about gastrointestinal applications of his technologies, including for evaluating esophageal cancer risk in patients with Barrett’s esophagus. Liu, who had a history of gastrointestinal cancer research as a postdoctoral researcher at Stanford and later as a junior investigator, was excited to get back to tackling gastrointestinal problems. Besides Reddi, other collaborators include William Grady, professor at the Fred Hutchinson Cancer Center; and Faisal Mahmood, associate professor of pathology at the Brigham and Women’s Hospital of Harvard Medical School.
“The goal is to show that AI analysis of 3D pathology datasets acquired from whole endoscopic biopsies can improve our ability to determine which Barrett’s esophagus patients are most likely to progress to esophageal cancer and therefore require more aggressive treatments,” Liu says.
Barrett’s esophagus is a common condition in which the cells that line the esophagus are altered due to chronic gastroesophageal reflux. People with Barrett’s esophagus have a small but heightened chance of developing esophageal cancer and are encouraged to undergo regular surveillance endoscopies to monitor for progression to pre-cancer or cancer.
Improving the standard of care
To monitor Barrett’s esophagus patients through surveillance endoscopies, gastroenterologists take dozens of biopsies from Barrett's esophagus tissues, which are then cut into thin sections that are mounted onto glass slides for microscopic examination by pathologists. However, the tissue sections viewed by pathologists represent 1% or less of the actual biopsies, and offer only a 2D view, which can be misleading.
Liu’s team aims to improve risk stratification of Barrett’s esophagus patients using open-top light-sheet microscopy, a method that allows biopsy specimens to be microscopically viewed in 3D without having to cut or destroy any of the tissue. This “slide-free” microscopy device uses a sheet of light and high-speed cameras to perform 3D imaging of tissue samples that are stained with fluorescent dyes and “optically cleared” to make them transparent to light. Once the 3D pathology datasets are generated, the team can use AI to triage the large datasets to find the most important parts of the biopsy to show the pathologist, or to automatically diagnose the tissues.
“We are trying to identify the highest risk patients so that they may receive early treatments that could be critical for their survival,” Liu says. “In our archived tissue samples, some patients progressed to cancer, and we are trying to detect what in their tissues could have predicted that at the earliest stages.”
In a previous study on AI-triaged 3D pathology published in Modern Pathology, the researchers developed a deep learning-based strategy that was more effective at detecting malignancies in Barrett’s esophagus biopsies than the current standard of care while reducing pathologists’ workloads by requiring them to review fewer images.
“3D pathology with AI promises to enhance prognosis accuracy for Barrett's esophagus patients while reducing pathologists’ workloads, creating a win-win situation.”
Gan Gao, ME Ph.D. student and researcher in Liu’s lab, co-wrote the paper with Lindsey Barner (ME Ph.D., ’22) and will be involved in the NIH-funded research. To make it more efficient to teach the AI model to recognize different tissue morphologies, Gao is developing an improved weakly-supervised deep learning triage pipeline for 3D pathology datasets.
“I’m particularly excited about the potential impact this project could have on clinical practice,” Gao says. “3D pathology with AI promises to enhance prognosis accuracy for Barrett's esophagus patients while reducing pathologists’ workloads, creating a win-win situation. Looking ahead, I look forward to exploring various computational approaches in 3D pathology to improve outcomes for Barrett’s esophagus patients at an early stage.”
For a fully computational approach towards risk stratification, a recent paper published in Cell detailed how a deep-learning platform for 3D pathology outperformed 2D AI models and standard-of-care methods for predicting clinical outcomes. The paper was co-authored by Liu and Mahmood along with ME Ph.D. students Sarah Chow, Gao, and many others.
“With AI-triaged 3D pathology, pathologists can still make the final call, but deep learning helps to quickly guide them to the right parts of the biopsy,” Liu says. “This method can potentially be adopted into clinics more quickly than a fully computational approach, but we believe that both approaches have value for the future adoption of non-destructive 3D pathology by clinicians.”
Research reported in this publication was supported by the National Institute of Diabetes and Digestive and Kidney Diseases of the National Institutes of Health under Award Number R01DK138948. The content is solely the responsibility of the authors and does not necessarily represent the official views of the National Institutes of Health.